The Uncomfortable Truth About AI and Sexual Objectification: A Deep Dive into Lensa AI
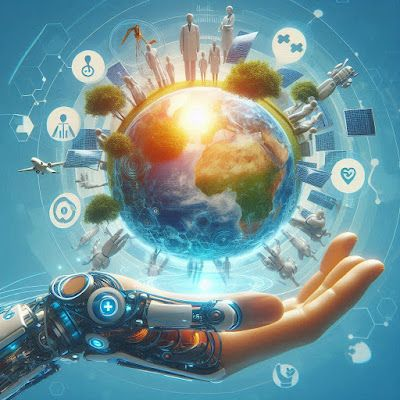
Recently, the release of Lensa AI, an app generating AI avatars, has sparked both fascination and concern. While many users enjoyed creative and flattering results, a darker side emerged – one highlighting the unsettling reality of AI and sexual objectification.
This article delves into the experiences of those who faced unwanted sexualization from Lensa AI, examining the underlying reasons and potential consequences of this bias. We’ll also explore the broader implications for AI development and the urgent need for ethical considerations.
Lensa AI: When AI Crosses the Line
Lensa AI utilizes Stable Diffusion, an open-source AI model trained on a massive dataset of images scraped from the internet. While this allows for diverse and impressive image generation, it also exposes a significant flaw: inherent biases within the data itself.
The internet, unfortunately, is saturated with objectified images of women. This overrepresentation seeps into the training data, leading AI models like Stable Diffusion to reproduce and amplify these harmful stereotypes.
Melissa Heikkilä, a writer at MIT Technology Review, shared her unsettling experience with Lensa AI. While colleagues received artistic and realistic avatars, Heikkilä, an Asian woman, was bombarded with sexualized images, many depicting her nude or in revealing attire.
This stark contrast highlights the deeply ingrained biases within AI models. Heikkilä’s experience wasn’t unique. Numerous users, particularly women, reported similar encounters with Lensa AI, receiving an overwhelming number of sexualized avatars despite providing regular photos.
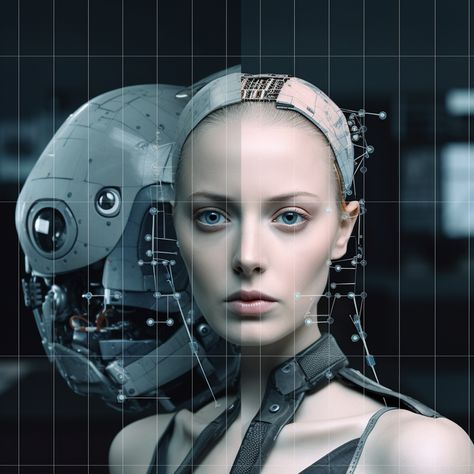
Unpacking the Roots of AI Bias
The issue extends beyond Lensa AI. It points to a systemic problem within AI development: the datasets used to train these models.
- Skewed Datasets: As mentioned earlier, datasets scraped from the internet often contain biased and stereotypical representations of gender, race, and other demographics. This skewed data directly influences the output of AI models.
- Lack of Diversity in AI Development: The lack of diversity within the teams developing and training these AI models further exacerbates the problem. A more diverse team can identify and address potential biases more effectively.
- Profit Over Ethics: The rush to release new and exciting AI tools often overshadows ethical considerations. Companies may prioritize profitability over addressing potential harms, leading to the deployment of biased and potentially harmful AI systems.
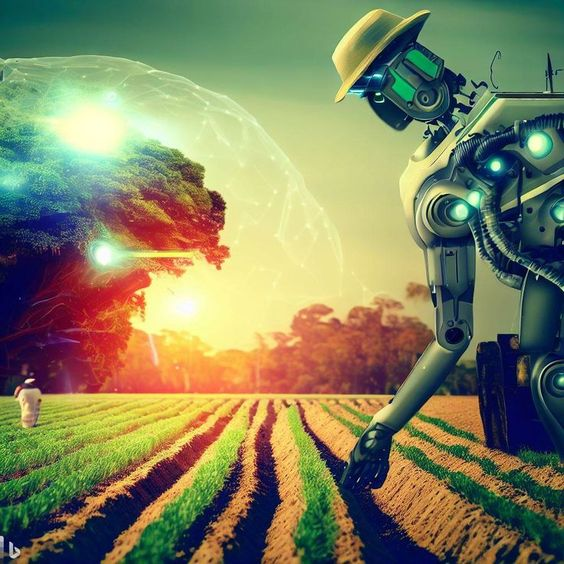
The Long-Term Consequences of AI Bias
The implications of biased AI extend far beyond a few unsettling avatars. These biases can have real-world consequences, perpetuating harmful stereotypes and impacting individuals and communities.
- Reinforcing Harmful Stereotypes: When AI models consistently generate sexualized images of women or depict specific racial groups in negative ways, it reinforces existing harmful stereotypes.
- Discrimination and Exclusion: Biased AI can lead to discrimination in various domains. For example, AI-powered hiring tools trained on biased data might unfairly disadvantage certain demographics.
- Erosion of Trust: As AI becomes increasingly integrated into our lives, instances of bias erode public trust in these technologies. This lack of trust can hinder the development and adoption of beneficial AI applications.
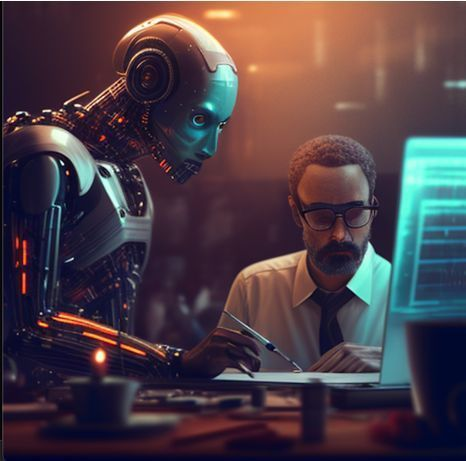
The Path Forward: Building Ethical and Inclusive AI
Addressing AI bias requires a multi-pronged approach involving developers, policymakers, and the tech community as a whole.
- Diverse and Inclusive Datasets: Creating and utilizing diverse and representative datasets is crucial to mitigate bias in AI models. This includes actively seeking out data that challenges existing stereotypes and accurately reflects the real world.
- Ethical Frameworks and Regulations: Developing clear ethical guidelines and regulations for AI development and deployment is essential. These frameworks should address issues of bias, fairness, and accountability.
- Transparency and Explainability: Building transparent and explainable AI models is crucial to understand how these systems make decisions and identify potential biases.
- Education and Awareness: Raising awareness about AI bias among developers, users, and the general public is essential. This includes promoting digital literacy and critical thinking skills to identify and challenge biased AI outputs.
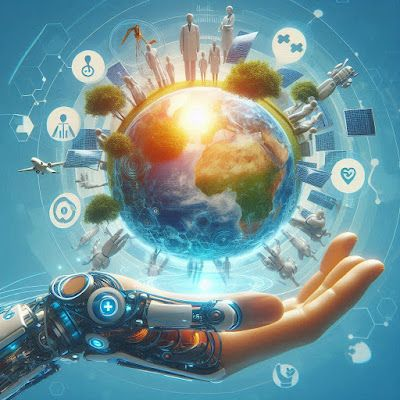
Resources for Further Exploration
- The Algorithm: MIT Technology Review’s weekly newsletter on AI, covering the latest developments and ethical considerations.
- Hugging Face: An AI community and platform providing tools and resources for ethical AI development.
- Partnership on AI: A multi-stakeholder organization dedicated to the responsible development and use of artificial intelligence.
The Lensa AI controversy serves as a stark reminder of the ethical challenges surrounding AI development. As we continue to push the boundaries of this powerful technology, we must prioritize building ethical, inclusive, and unbiased AI systems that benefit all of humanity.