The Dark Side of Viral AI Art: Why Lensa’s “Magic Avatars” Are Raising Red Flags
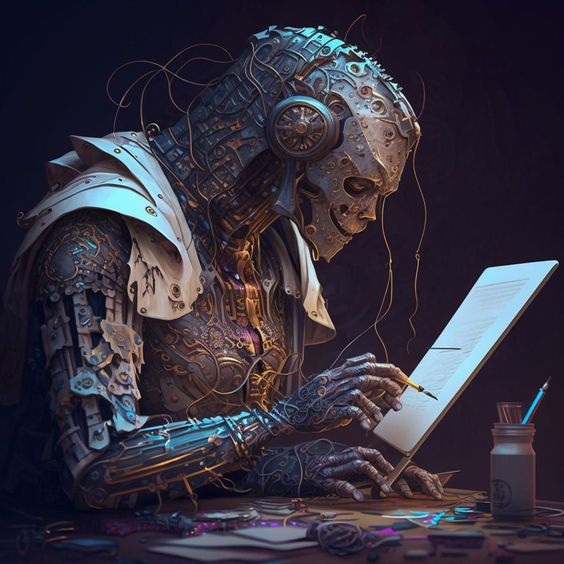
The world of AI image generation is exploding, with apps like Lensa captivating users with the promise of transforming selfies into stunning digital art. But behind the whimsical filters and stylized portraits lurks a darker side – one rife with ethical concerns and the potential for harm. This deep dive explores the controversial reality of Lensa’s “Magic Avatars” feature, examining its inherent biases, the dangers of unregulated AI, and the urgent need for responsible development in this rapidly evolving field.
The Dark Side of Viral AI Art: Why Lensa’s “Magic Avatars” Are Raising Red Flags
The tech world is buzzing with excitement over AI image generators, and leading the charge is Lensa. This app has taken social media by storm with its “Magic Avatars” feature, allowing users to transform their selfies into fantastical digital portraits. But beneath the surface of this viral sensation lies a deeply concerning issue: the perpetuation of harmful biases that disproportionately impact women and marginalized communities.
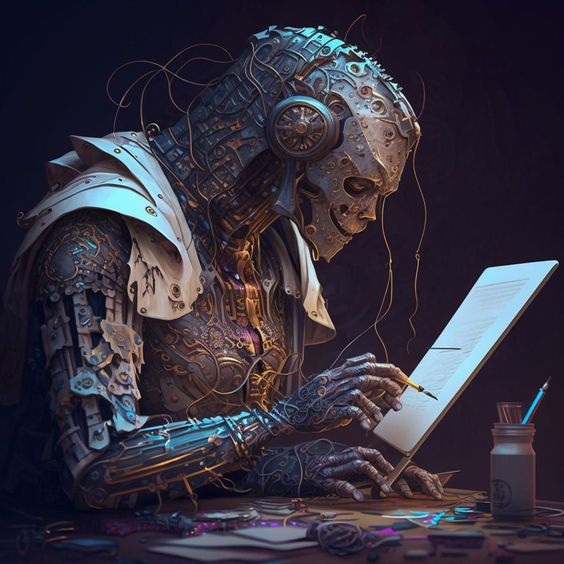
A Disturbing Trend Emerges:
As users flocked to Lensa, a disturbing trend began to emerge. While some delighted in their AI-generated avatars, many women found themselves subjected to a barrage of hypersexualized and racially insensitive imagery. Reports flooded in of women receiving avatars depicting them in overtly sexualized poses, often scantily clad or entirely nude, even when their input photos were innocuous. This issue was particularly pronounced for women of Asian descent, highlighting the deeply ingrained biases within the technology.
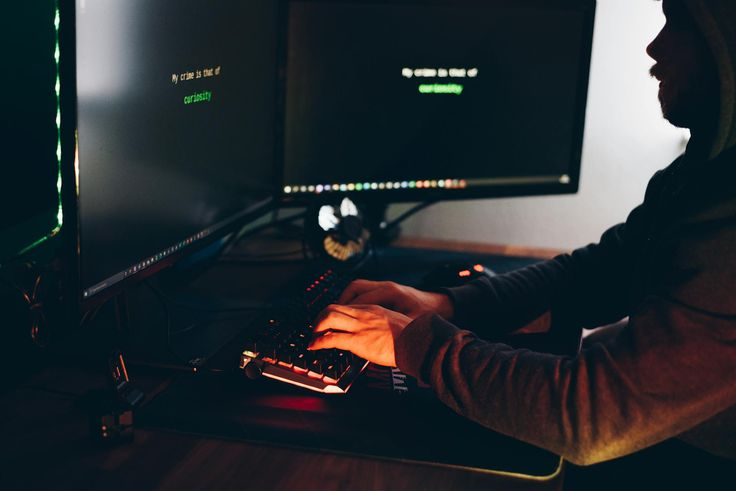
Unveiling the Root of the Problem: Biased Data, Biased Output:
At the heart of Lensa’s “Magic Avatars” lies Stable Diffusion, a powerful AI model trained on a massive dataset of images and text scraped from the internet. This dataset, known as LAION-5B, forms the foundation of the AI’s understanding of the world and its ability to generate images. However, this vast collection of data is far from neutral. It reflects the biases and prejudices that permeate the online world, including a disproportionate amount of pornography and stereotypical representations of women and minorities.
Understanding the Mechanics of AI Image Generation:
To grasp the full extent of the problem, it’s crucial to understand how AI image generators like Stable Diffusion actually work. These models learn by analyzing millions of images and their corresponding text descriptions, identifying patterns and associations. This process, known as “training,” enables the AI to generate new images based on text prompts or, in Lensa’s case, user-uploaded selfies. However, when the training data itself is skewed, the AI’s output will inevitably reflect those biases.

Lensa is Not Alone: A Sector-Wide Challenge:
While Lensa has come under fire for its biased outputs, it’s important to recognize that this is not an isolated incident. The issue of bias plagues the entire field of AI image generation. Other popular tools like OpenAI’s DALL-E and Google’s Imagen, while not immune to these challenges, operate with significantly less transparency. Access to their training data is often restricted, making it difficult to assess and address potential biases.
The Ethical Imperative: Consent, Representation, and Harm Reduction:
The implications of biased AI image generation extend far beyond mere technological shortcomings. These tools raise serious ethical concerns, particularly regarding consent, representation, and the potential for harm. The non-consensual generation of sexualized and exploitative imagery can have a profound impact on individuals, perpetuating harmful stereotypes and contributing to a culture of objectification. This is particularly concerning given the potential for misuse, such as the creation of non-consensual deepfakes.
Towards a More Responsible Future for AI Art:
The rapid advancement of AI image generation necessitates a parallel focus on ethical development and responsible use. Developers have a moral obligation to address the biases inherent in training data and implement safeguards to mitigate potential harm. This includes:
- Curating Diverse and Representative Datasets: Moving away from vast, unfiltered datasets like LAION-5B and towards more carefully curated collections that reflect the diversity of human experiences.
- Developing Robust Bias Detection Tools: Investing in sophisticated tools and techniques to identify and mitigate biases in both training data and AI outputs.
- Establishing Ethical Guidelines and Industry Standards: Fostering collaboration within the AI community to establish clear ethical guidelines and industry standards for responsible AI development and deployment.
The Future of AI Art: A Balancing Act:
The future of AI art hinges on our ability to balance the immense potential of this technology with the responsibility to use it ethically. By acknowledging the limitations of current approaches, investing in bias mitigation strategies, and fostering a culture of transparency and accountability, we can harness the power of AI to create a more inclusive and equitable digital landscape.
Resources: